Drew Paine
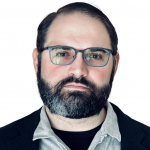
Drew Paine is a UX Researcher with more than a decade of experience in scientific and cloud computing UX.
He received his PhD from the Department of Human Centered Design & Engineering (HCDE) at the University of Washington in Seattle, WA in August 2016. Working in Dr. Charlotte Lee's Computer Supported Collaboration (CSC) Laboratory, Drew investigated the collaborative work of scientists in physics & astronomy, microbiology, oceanography, and climate science. He received a Software Engineering BS from Rose-Hulman Institute of Technology in May 2010.
Journal Articles
Devarshi Ghoshal, Ludovico Bianchi, Abdelilah Essiari, Michael Beach, Drew Paine, Lavanya Ramakrishnan, "Science Capsule - Capturing the Data Life Cycle", Journal of Open Source Software, 2021, 6:2484, doi: 10.21105/joss.02484
Marco Pritoni, Drew Paine, Gabriel Fierro, Cory Mosiman, Michael Poplawski, Joel Bender, Jessica Granderson, "Metadata Schemas and Ontologies for Building Energy Applications: A Critical Review and Use Case Analysis", Energies, April 6, 2021, doi: 10.3390/en14072024
Digital and intelligent buildings are critical to realizing efficient building energy operations and a smart grid. With the increasing digitalization of processes throughout the life cycle of buildings, data exchanged between stakeholders and between building systems have grown significantly. However, a lack of semantic interoperability between data in different systems is still prevalent and hinders the development of energy-oriented applications that can be reused across buildings, limiting the scalability of innovative solutions. Addressing this challenge, our review paper systematically reviews metadata schemas and ontologies that are at the foundation of semantic interoperability necessary to move toward improved building energy operations. The review finds 40 schemas that span different phases of the building life cycle, most of which cover commercial building operations and, in particular, control and monitoring systems. The paper’s deeper review and analysis of five popular schemas identify several gaps in their ability to fully facilitate the work of a building modeler attempting to support three use cases: energy audits, automated fault detection and diagnosis, and optimal control. Our findings demonstrate that building modelers focused on energy use cases will find it difficult, labor intensive, and costly to create, sustain, and use semantic models with existing ontologies. This underscores the significant work still to be done to enable interoperable, usable, and maintainable building models. We make three recommendations for future work by the building modeling and energy communities: a centralized repository with a search engine for relevant schemas, the development of more use cases, and better harmonization and standardization of schemas in collaboration with industry to facilitate their adoption by stakeholders addressing varied energy-focused use cases.
Drew Paine, Devarshi Ghoshal, Lavanya Ramakrishnan, "Experiences with a Flexible User Research Process to Build Data Change Tools", Journal of Open Research Software, September 1, 2020, doi: 10.5334/jors.284
Scientific software development processes are understood to be distinct from commercial software development practices due to uncertain and evolving states of scientific knowledge. Sustaining these software products is a recognized challenge, but under-examined is the usability and usefulness of such tools to their scientific end users. User research is a well-established set of techniques (e.g., interviews, mockups, usability tests) applied in commercial software projects to develop foundational, generative, and evaluative insights about products and the people who use them. Currently these approaches are not commonly applied and discussed in scientific software development work. The use of user research techniques in scientific environments can be challenging due to the nascent, fluid problem spaces of scientific work, varying scope of projects and their user communities, and funding/economic constraints on projects.
In this paper, we reflect on our experiences undertaking a multi-method user research process in the Deduce project. The Deduce project is investigating data change to develop metrics, methods, and tools that will help scientists make decisions around data change. There is a lack of common terminology since the concept of systematically measuring and managing data change is under explored in scientific environments. To bridge this gap we conducted user research that focuses on user practices, needs, and motivations to help us design and develop metrics and tools for data change. This paper contributes reflections and the lessons we have learned from our experiences. We offer key takeaways for scientific software project teams to effectively and flexibly incorporate similar processes into their projects.
Drew Paine, Charlotte P. Lee, "Coordinative Entities: Forms of Organizing in Data Intensive Science", Journal of Computer Supported Cooperative Work, February 11, 2020, doi: 10.1007/s10606-020-09372-2
Conference Papers
Devarshi Ghoshal, Ludovico Bianchi, Abdelilah Essiari, Drew Paine, Sarah Poon, Michael Beach, Alpha N'Diaye, Patrick Huck, Lavanya Ramakrishnan, "Science Capsule: Towards Sharing and Reproducibility of Scientific Workflows", 2021 IEEE Workshop on Workflows in Support of Large-Scale Science (WORKS), November 15, 2021, doi: 10.1109/WORKS54523.2021.00014
Workflows are increasingly processing large volumes of data from scientific instruments, experiments and sensors. These workflows often consist of complex data processing and analysis steps that might include a diverse ecosystem of tools and also often involve human-in-the-loop steps. Sharing and reproducing these workflows with collaborators and the larger community is critical but hard to do without the entire context of the workflow including user notes and execution environment. In this paper, we describe Science Capsule, which is a framework to capture, share, and reproduce scientific workflows. Science Capsule captures, manages and represents both computational and human elements of a workflow. It automatically captures and processes events associated with the execution and data life cycle of workflows, and lets users add other types and forms of scientific artifacts. Science Capsule also allows users to create `workflow snapshots' that keep track of the different versions of a workflow and their lineage, allowing scientists to incrementally share and extend workflows between users. Our results show that Science Capsule is capable of processing and organizing events in near real-time for high-throughput experimental and data analysis workflows without incurring any significant performance overheads.
Devarshi Ghoshal, Drew Paine, Gilberto Pastorello, Abdelrahman Elbashandy, Dan Gunter, Oluwamayowa Amusat, Lavanya Ramakrishnan, "Experiences with Reproducibility: Case Studies from Scientific Workflows", (P-RECS'21) Proceedings of the 4th International Workshop on Practical Reproducible Evaluation of Computer Systems, ACM, June 21, 2021, doi: 10.1145/3456287.3465478
Reproducible research is becoming essential for science to ensure transparency and for building trust. Additionally, reproducibility provides the cornerstone for sharing of methodology that can improve efficiency. Although several tools and studies focus on computational reproducibility, we need a better understanding about the gaps, issues, and challenges for enabling reproducibility of scientific results beyond the computational stages of a scientific pipeline. In this paper, we present five different case studies that highlight the reproducibility needs and challenges under various system and environmental conditions. Through the case studies, we present our experiences in reproducing different types of data and methods that exist in an experimental or analysis pipeline. We examine the human aspects of reproducibility while highlighting the things that worked, that did not work, and that could have worked better for each of the cases. Our experiences capture a wide range of scenarios and are applicable to a much broader audience who aim to integrate reproducibility in their everyday pipelines.
Christine T. Wolf, Drew Paine, "Sensemaking Practices in the Everyday Work of AI/ML Software Engineering", IEEE/ACM 42nd International Conference on Software Engineering Workshops (ICSEW’20), ACM, April 5, 2020, doi: 10.1145/3387940.3391496
Christine T. Wolf, Julia Bullard, Stacy Wood, Amelia Acker, Drew Paine, Charlotte P. Lee, "Mapping the “How” of Collaborative Action: Research Methods for Studying Contemporary Sociotechnical Processes", CSCW '19: Conference Companion Publication of the 2019 on Computer Supported Cooperative Work and Social Computing, November 10, 2019, doi: 10.1145/3311957.3359441
Process has been a concern since the beginning of CSCW. Developments in sociotechnical landscapes raise new challenges for studying processes (e.g., massive online communities bringing together vast crowds; Big Data technologies connecting many through the flow of data). This re-opens questions about how we study, document, conceptualize, and design to support processes in complex, contemporary sociotechnical systems. This one-day workshop will bring together researchers to discuss the CSCW community’s unique focus and methodological toolkit for studying process and workflow; provide a collaborative space for the improvement and extension of research projects within this space; and catalyze a network of scholars with expertise and interest in addressing challenging methodological questions around studying process in contemporary, sociotechnical systems.
Drew Paine, Lavanya Ramakrishnan, "Surfacing Data Change in Scientific Work", iConference 2019, Springer Verlag, March 19, 2019, 15-26, doi: 10.1007/978-3-030-15742-5_2
David P. Randall, Drew Paine, Charlotte P. Lee, "Educational Outreach & Stakeholder Role Evolution in a Cyberinfrastructure Project", 2018 IEEE 14th International Conference on e-Science, IEEE Computer Society, 2018, 201-211, doi: 10.1109/eScience.2018.00035
Presentation/Talks
Cheah You-Wei, Drew Paine, Devarshi Ghoshal, Lavanya Ramakrishnan, Bringing Data Science to Qualitative Analysis, 2018 IEEE 14th International Conference on e-Science, Pages: 325-326 2018, doi: 10.1109/eScience.2018.00076
Reports
Drew Paine, Sarah Poon, Lavanya Ramakrishnan, "Investigating User Experiences with Data Abstractions on High Performance Computing Systems", June 29, 2021, LBNL LBNL-2001374,
Scientific exploration generates expanding volumes of data that commonly require High Performance Computing (HPC) systems to facilitate research. HPC systems are complex ecosystems of hardware and software that frequently are not user friendly. The Usable Data Abstractions (UDA) project set out to build usable software for scientific workflows in HPC environments by undertaking multiple rounds of qualitative user research. Qualitative research investigates how individuals accomplish their work and our interview-based study surfaced a variety of insights about the experiences of working in and with HPC ecosystems. This report examines multiple facets to the experiences of scientists and developers using and supporting HPC systems. We discuss how stakeholders grasp the design and configuration of these systems, the impacts of abstraction layers on their ability to successfully do work, and the varied perceptions of time that shape this work. Examining the adoption of the Cori HPC at NERSC we explore the anticipations and lived experiences of users interacting with this system's novel storage feature, the Burst Buffer. We present lessons learned from across these insights to illustrate just some of the challenges HPC facilities and their stakeholders need to account for when procuring and supporting these essential scientific resources to ensure their usability and utility to a variety of scientific practices.
Drew Paine, Lavanya Ramakrishnan, "Understanding Interactive and Reproducible Computing With Jupyter Tools at Facilities", LBNL Technical Report, October 31, 2020, LBNL LBNL-2001355,
Increasingly Jupyter tools are being adopted and incorporated into High Performance Computing (HPC) and scientific user facilities. Adopting Jupyter tools enables more interactive and reproducible computational work at facilities across data life cycles. As the volume, variety, and scope of data grow, scientists need to be able to analyze and share results in user friendly ways. Human-centered research highlights design challenges around computational notebooks, and our qualitative user study shifts focus to better characterize how Jupyter tools are being used in HPC and science user facilities today. We conducted twenty-nine interviews, and obtained 103 survey responses from NERSC Jupyter users, to better understand the increasing role of interactive computing tools in DOE sponsored scientific work. We examine a range of issues that emerge using and supporting Jupyter in HPC ecosystems, including: how Jupyter is being used by scientists in HPC and user facility ecosystems; how facilities are purposefully supporting Jupyter in their ecosystems; feedback NERSC users have about the facility’s deployment, and, discuss features NERSC indicated would be helpful. We offer a variety of takeaways for staff supporting Jupyter at facilities, Project Jupyter and related open source communities, and funding agencies supporting interactive computing work.
Drew Paine, Devarshi Ghoshal, Lavanya Ramakrishnan, "Investigating Scientific Data Change with User Research Methods", August 20, 2020, LBNL LBNL-2001347,
Scientific datasets are continually expanding and changing due to fluctuations with instruments, quality assessment and quality control processes, and modifications to software pipelines. Datasets include minimal information about these changes or their effects requiring scientists manually assess modifications through a number of labor intensive and ad-hoc steps. The Deduce project is investigating data change to develop metrics, methods, and tools that will help scientists systematically identify and make decisions around data changes. Currently, there is a lack of understanding, and common practices, for identifying and evaluating changes in datasets since systematically measuring and managing data change is under explored in scientific work. We are conducting user research to address this need by exploring scientist's conceptualizations, behaviors, needs, and motivations when dealing with changing datasets. Our user research utilizes multiple methods to produce foundational, generative insights and evaluate research products produced by our team. In this paper, we detail our user research process and outline our findings about data change that emerge from our studies. Our work illustrates how scientific software teams can push beyond just usability testing user interfaces or tools to better probe the underlying ideas they are developing solutions to address.