Oluwamayowa (Mayo) Amusat
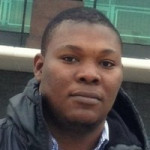
Oluwamayowa (Mayo) Amusat is a computational research scientist working on the application of optimization and machine learning techniques to the design of advanced energy, water and scientific systems.
Oluwamayowa's research interests centre around the development of numerical optimization, machine learning, PSE, and decision-support tools for the enhancement and improvement of scientific and engineering systems. Oluwamayowa is part of the IDAES, NAWI/WaterTAP, PROMMIS and ScienceSearch projects.
Oluwamayowa originally joined Berkeley Lab in February 2019 as a post-doctoral scholar. He received his PhD in Chemical Engineering from University College London (UCL).
» Visit Oluwamayowa Amusat's personal web page
Journal Articles
Oluwamayowa O Amusat, Harshad Hegde, Christopher J Mungall, Anna Giannakou, Neil P Byers, Dan Gunter, Kjiersten Fagnan, Lavanya Ramakrishnan, "Automated annotation of scientific texts for ML-based keyphrase extraction and validation", Database, September 27, 2024, 2024:baae093, doi: https://doi.org/10.1093/database/baae093
Oluwamayowa O Amusat, Adam A Atia, Alexander V Dudchenko, Timothy V Bartholomew, "Modeling Framework for Cost Optimization of Process-Scale Desalination Systems with Mineral Scaling and Precipitation", ACS ES&T Engineering, March 8, 2024, doi: 10.1021/acsestengg.3c00537
Mohammed A. Alhussaini, Zachary M. Binger, Bianca M. Souza-Chaves, Oluwamayowa O. Amusat, Jangho Park, Timothy V. Bartholomew, Dan Gunter, Andrea Achilli, "Analysis of backwash settings to maximize net water production in an engineering-scale ultrafiltration system for water reuse", Journal of Water Process Engineering, 2023, 53, doi: 10.1016/j.jwpe.2023.103761
Conference Papers
S. Burroughs, B. Lincoln, A. Adeel, I. Severinsen, A. Lee, O. Amusat, D. Gunter, B. Nicholson, M. Apperley, B. Young, J. Siirola, T. G. Walmsley, "New Directions and Software Tools Within the Process Systems Engineering Ecosystem", Systems and Control Transactions, Ghent, Belgium, PSE Press: Hamilton, July 1, 2025, 4:430-436, doi: https://doi.org/10.69997/sct.156838
Chris A. Laliwala, Oluwamayowa O. Amusat, Ana I. Torres, "An Optimization-Based Law of Mass Action Precipitation/Dissolution Model", Systems and Control Transactions, Ghent, Belgium, PSE Press: Hamilton, July 1, 2025, 4:2140-2145, doi: https://doi.org/10.69997/sct.132742
Jordan A. Welsman, Gunther H. Weber, Oluwamayowa O. Amusat, Anna Giannakou, Lavanya Ramakrishnan, "Enhancing Electron Microscopy Image Classification Using Data Augmentation", SC24-W: Workshops of the International Conference for High Performance Computing, Networking, Storage and Analysis, IEEE, November 17, 2024, 64-71, doi: 10.1109/SCW63240.2024.00016
Alexander V. Dudchenko, Oluwamayowa O. Amusat, "Neural Networks for Prediction of Complex Chemistry in Water Treatment Process Optimization", Proceedings of the 10th International Conference on Foundations of Computer-Aided Process Design (FOCAPD 2024), Denver, PSE Press, July 19, 2024, 3:267-274, doi: 10.69997/sct.107047
Oluwamayowa O. Amusat, Alexander V. Dudchenko, Adam A. Atia, Timothy Bartholomew, "Cost-optimal Selection of pH Control for Mineral Scaling Prevention in High Recovery Reverse Osmosis Desalination", Proceedings of the 10th International Conference on Foundations of Computer-Aided Process Design (FOCAPD 2024), Denver, PSE Press, July 9, 2024, 3:253-260, doi: 10.69997/sct.143335
Devarshi Ghoshal, Drew Paine, Gilberto Pastorello, Abdelrahman Elbashandy, Dan Gunter, Oluwamayowa Amusat, Lavanya Ramakrishnan, "Experiences with Reproducibility: Case Studies from Scientific Workflows", (P-RECS'21) Proceedings of the 4th International Workshop on Practical Reproducible Evaluation of Computer Systems, ACM, June 21, 2021, doi: 10.1145/3456287.3465478
Reproducible research is becoming essential for science to ensure transparency and for building trust. Additionally, reproducibility provides the cornerstone for sharing of methodology that can improve efficiency. Although several tools and studies focus on computational reproducibility, we need a better understanding about the gaps, issues, and challenges for enabling reproducibility of scientific results beyond the computational stages of a scientific pipeline. In this paper, we present five different case studies that highlight the reproducibility needs and challenges under various system and environmental conditions. Through the case studies, we present our experiences in reproducing different types of data and methods that exist in an experimental or analysis pipeline. We examine the human aspects of reproducibility while highlighting the things that worked, that did not work, and that could have worked better for each of the cases. Our experiences capture a wide range of scenarios and are applicable to a much broader audience who aim to integrate reproducibility in their everyday pipelines.