Bin Wang
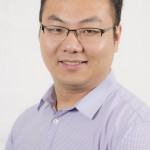
Biographical Sketch
Bin Wang is a research scientist in the Energy Technologies Area at Lawrence Berkeley National Laboratory, and a former postdoctoral researcher in the Computer Architecture Group in the Computational Research Division. His research interests include control and optimization strategies in the fields of power system, transportation system, electric vehicles, and internet of things, etc., and the integration of large-scale simulation with high performance computing platform. Bin received his Bachelor’s degree in vehicle engineering from Jilin University, China in 2012, and his Ph.D degree in mechanical engineering from University of California, Los Angeles in 2016.
Current Projects
- Mobiliti: Scalable Transportation Simulation Using High-Performance Computing
Journal Articles
Dai Wang, Junyu Gaob, Pan Li, Bin Wang, Cong Zhang, Samveg Saxena, "Modeling of plug-in electric vehicle travel patterns and charging load based on trip chain generation", Journal of Power Sources, May 13, 2017, 359:468 - 479, doi: 10.1016/j.jpowsour.2017.05.036
Modeling PEV travel and charging behavior is the key to estimate the charging demand and further explore the potential of providing grid services. This paper presents a stochastic simulation methodology to generate itineraries and charging load profiles for a population of PEVs based on real-world vehicle driving data. In order to describe the sequence of daily travel activities, we use the trip chain model which contains the detailed information of each trip, namely start time, end time, trip distance, start location and end location. A trip chain generation method is developed based on the Naive Bayes model to generate a large number of trips which are temporally and spatially coupled. We apply the proposed methodology to investigate the multi-location charging loads in three different scenarios. Simulation results show that home charging can meet the energy demand of the majority of PEVs in an average condition. In addition, we calculate the lower bound of charging load peak on the premise of lowest charging cost. The results are instructive for the design and construction of charging facilities to avoid excessive infrastructure.
Yubo Wan, Wenbo Shi, Bin Wang, Chi-Cheng Ch, Rajit Gadh, "Optimal operation of stationary and mobile batteries in distribution grids", Applied Energy, January 28, 2017, 190:1289 - 130, doi: 10.1016/j.apenergy.2016.12.139
The trending integrations of Battery Energy Storage System (BESS, stationary battery) and Electric Vehicles (EV, mobile battery) to distribution grids call for advanced Demand Side Management (DSM) technique that addresses the scalability concerns of the system and stochastic availabilities of EVs. Towards this goal, a stochastic DSM is proposed to capture the uncertainties in EVs. Numerical approximation is then used to make the problem tractable. To accelerate the computational speed, the proposed DSM is tightly relaxed to a convex form using second-order cone programming. Furthermore, in light of the continuous increasing problem size, a distributed method with a guaranteed convergence is applied to shift the centralized computational burden to distributed controllers. To verify the proposed DSM, real-life EV data collected on UCLA campus is used to test the proposed DSM in an IEEE benchmark test system. Numerical results demonstrate the correctness and merits of the proposed approach.
Bin Wang, Yubo Wang, Hamidreza Nazaripouya, Charlie Qiu, Chi-Cheng Chu, Rajit Gadh, "Predictive Scheduling Framework for Electric Vehicles with Uncertainties of User Behaviors", IEEE Internet of Things Journal, October 13, 2016, 4:52 - 63, doi: 10.1109/JIOT.2016.2617314
The randomness of user behaviors plays a significant role in electric vehicle (EV) scheduling problems, especially when the power supply for EV supply equipment (EVSE) is limited. Existing EV scheduling methods do not consider this limitation and assume charging session parameters, such as stay duration and energy demand values, are perfectly known, which is not realistic in practice. In this paper, based on real-world implementations of networked EVSEs on University of California at Los Angeles campus, we developed a predictive scheduling framework, including a predictive control paradigm and a kernel-based session parameter estimator. Specifically, the scheduling service periodically computes for cost-efficient solutions, considering the predicted session parameters, by the adaptive kernel-based estimator with improved estimation accuracies. We also consider the power sharing strategy of existing EVSEs and formulate the virtual load constraint to handle the future EV arrivals with unexpected energy demand. To validate the proposed framework, 20-fold cross validation is performed on the historical dataset of charging behaviors for over one-year period. The simulation results demonstrate that average unit energy cost per kWh can be reduced by 29.42% with the proposed scheduling framework and 66.71% by further integrating solar generations with the given capacity, after the initial infrastructure investment. The effectiveness of kernel-based estimator, virtual load constraint, and event-based control scheme are also discussed in detail.
Yubo Wang, Bin Wang, Chi-Cheng Chu, Hemanshu Pota, Rajit Gadh, "Energy management for a commercial building microgrid with stationary and mobile battery storage", Energy and Buildings, December 30, 2015, 116:141 - 150, doi: 10.1016/j.enbuild.2015.12.055
This paper investigates the Demand Side Management (DSM) in a commercial building microgrid with solar generation, stationary Battery Energy Management System (BESS) and gridable (V2G) Electric Vehicle (EV) integration. Taking into consideration of a comprehensive pricing model, we first formulate a deterministic DSM as a mixed integer linear programming problem, assuming perfect knowledge of the uncertainties in the system. A two-stage stochastic DSM is further developed that addresses the stochastic nature in solar generation, loads, EV availabilities and EV energy demands. The proposed DSMs are validated with real solar generation, loads, BESS and EV data using sample average approximation. Detailed case studies show that the stochastic DSM outperforms its deterministic counterpart for cost saving for a wide range of prices, though at the expense of higher computational time. Computational results also demonstrate that moderate number of EVs helps to cut down the overall operation cost, which sheds light on the benefit of future large scale EV integration to smart buildings.
Conference Papers
Serges Love Teutu Talla, Isabelle Kemajou-Brown, Cy Chan, Bin Wang, "A Binary Multi-Subsystems Transportation Networks Estimation using Mobiliti Data", 2021 American Control Conference (ACC), May 25, 2021,
Bin Wang, Dai Wang, Rongxin Yin, Doug Black, Cy Chan, "Predictive management of electric vehicles in a community microgrid", 2020 IEEE Transportation Electrification Conference & Expo (ITEC), June 23, 2020,
Bin Wang, Cy Chan, Divya Somasi, Jane Macfarlane, Eric Rask, "Data-Driven Energy Use Estimation in Large Scale Transportation Networks", Proceedings of the 2nd ACM/EIGSCC Symposium on Smart Cities and Communities - SCC '19, ACM Press, September 10, 2019,
Cy P Chan, Bin Wang, John D Bachan, Jane Macfarlane, "Mobiliti: Scalable Transportation Simulation Using High-Performance Parallel Computing", 2018 IEEE International Conference on Intelligent Transportation Systems (ITSC), November 6, 2018,
- Download File: ITSC18-0935-MS-Final.pdf (pdf: 1.4 MB)
Bin Wang, John D Bachan, Cy P Chan, "ExaGridPF: A parallel power flow solver for transmission and unbalanced distribution systems", 2018 IEEE Power & Energy Society Innovative Smart Grid Technologies Conference (ISGT), February 22, 2018,
Yingqi Xiong, Bin Wang, Chi-cheng Chu, Rajit Gadh, "Distributed Optimal Vehicle Grid Integration Strategy with User Behavior Prediction", IEEE PES General Meeting 2017, March 13, 2017,
With the increasing of electric vehicle (EV) adoption in recent years, the impact of EV charging activities to the power grid becomes more and more significant. In this article, an optimal scheduling algorithm which combines smart EV charging and V2G gird service is developed to integrate EVs into power grid as distributed energy resources, with improved system cost performance. Specifically, an optimization problem is formulated and solved at each EV charging station according to control signal from aggregated control center and user charging behavior prediction by mean estimation and linear regression. The control center collects distributed optimization results and updates the control signal, periodically. The iteration continues until it converges to optimal scheduling. Experimental result shows this algorithm helps fill the valley and shave the peak in electric load profiles within a microgrid, while the energy demand of individual driver can be satisfied.
Yingqi Xiong, Bin Wang, Zhiyuan Cao, Chi-cheng Chu, Hemanshu Pota, Rajit Gadh, "Extension of IEC61850 with smart EV charging", Innovative Smart Grid Technologies - Asia (ISGT-Asia), 2016 IEEE, Melbourne, VIC, Australia, IEEE, December 26, 2016, 294 - 299, doi: 10.1109/ISGT-Asia.2016.7796401
Un-coordinated Electric Vehicle (EV) charging behaviors will impact the power grid and degrade power quality. Smart charging system can optimally allocate the energy among EVs and minimize the impact on grid. IEC 61850 is an international standard for distribution & substation automation and intelligence device communication in smart grid. This paper introduces the extension of IEC 61850 with smart EV scheduling algorithms, considering current multiplexing, power sharing strategies and user behaviors. We have developed an IEC 61850 abstract data model for the information exchanged among components of smart EV charging infrastructures, including the EV charging control center, intelligent Electric Vehicle Supply Equipment (EVSE) and the EV users with mobile applications. Real-world EV usage data on UCLA campus is utilized in the simulation experiment, which is based on the predictive control paradigm. The data model is instantiated by a web service on EV control center by converting the raw data streams from EVSEs in various formats, such as JSON or raw string, etc. into a standardized IEC 61850 SCL file, which contains the critical meter data and charging session parameters. The system cost performance, the interoperability and scalability of smart EV charging infrastructure are greatly improved.
H. Nazaripouya, B. Wang, Y. Wang, P. Chu, H. R. Pota, R. Gadh, "Univariate Time Series Prediction of Solar Power Using a Hybrid Wavelet-ARMA-NARX Prediction Method", 2016 IEEE/PES Transmission and Distribution Conference and Exposition (T&D), Dallas, TX, USA, IEEE, July 25, 2016, 1 - 5, doi: 10.1109/TDC.2016.7519959
This paper proposes a new hybrid method for super short-term solar power prediction. Solar output power usually has a complex, nonstationary, and nonlinear characteristic due to intermittent and time varying behavior of solar radiance. In addition, solar power dynamics is fast and is inertia less. An accurate super short-time prediction is required to compensate for the fluctuations and reduce the impact of solar power penetration on the power system. The objective is to predict one step-ahead solar power generation based only on historical solar power time series data. The proposed method incorporates discrete wavelet transform (DWT), Auto-Regressive Moving Average (ARMA) models, and Recurrent Neural Networks (RNN), while the RNN architecture is based on Nonlinear Auto-Regressive models with eXogenous inputs (NARX). The wavelet transform is utilized to decompose the solar power time series into a set of richer-behaved forming series for prediction. ARMA model is employed as a linear predictor while NARX is used as a nonlinear pattern recognition tool to estimate and compensate the error of wavelet-ARMA prediction. The proposed method is applied to the data captured from UCLA solar PV panels and the results are compared with some of the common and most recent solar power prediction methods. The results validate the effectiveness of the proposed approach and show a considerable improvement in the prediction precision.
Yubo Wang, Bin Wang, Tianyang Zhang, Hamidreza Nazaripouya, Chi-Cheng Chu, Rajit Gadh, "Optimal Energy Management for Microgrid with Stationary and Mobile Storages", 2016 IEEE/PES Transmission and Distribution Conference and Exposition (T&D), Dallas, TX, USA, IEEE, July 25, 2016, 1 - 5, doi: 10.1109/TDC.2016.7520004
This paper studies energy management in a Microgrid (MG) with solar generation, Battery Energy Management System (BESS) and gridable (V2G) Electric Vehicles (EVs). A two-stage stochastic optimization method is proposed to capture the intermittent solar generation and random EV user behaviors. It is subsequently formulated as a Mixed Integer Linear Programming (MILP) problem. To evaluate the proposed method, real solar generation, loads, BESS and EV data is used in Sample Average Approximation (SAA). Computational results show the correctness of the proposed method as well as steady and tightly bounded optimality gap. Comparisons demonstrate that the proposed stochastic method outperforms its deterministic counterpart at the expense of higher computational cost. It is also observed that moderate number of EVs helps to reduce the overall operational cost of the MG, which sheds light on future EV integration to the smart grid.
Yubo Wang, Bin Wang, Rui Huang, Chi-Cheng Chu, Hemanshu R. Pota, Rajit Gadh, "Two-Tier Prediction of Solar Power Generation with Limited Sensing Resource", 2016 IEEE/PES Transmission and Distribution Conference and Exposition (T&D), Dallas, TX, USA, IEEE, July 25, 2016, 1 - 5, doi: 10.1109/TDC.2016.7519968
This paper considers a typical solar installations scenario with limited sensing resources. In the literature, there exist either day-ahead solar generation prediction methods with limited accuracy, or high accuracy short timescale methods that are not suitable for applications requiring longer term prediction. We propose a two-tier (global-tier and local-tier) prediction method to improve accuracy for long term (24 hour) solar generation prediction using only the historical power data. In global-tier, we examine two popular heuristic methods: weighted k-Nearest Neighbors (k-NN) and Neural Network (NN). In local-tier, the global-tier results are adaptively updated using real-time analytical residual analysis. The proposed method is validated using the UCLA Microgrid with 35kW of solar generation capacity. Experimental results show that the proposed two-tier prediction method achieves higher accuracy compared to day-ahead predictions while providing the same prediction length. The difference in the overall prediction performance using either weighted k-NN based or NN based in the global-tier are carefully discussed and reasoned. Case studies with a typical sunny day and a cloudy day are carried out to demonstrate the effectiveness of the proposed two-tier predictions.
Bin Wang, Rui Huang, Yubo Wang, Hamidreza Nazaripouya, Charlie Qiu, Chi-Cheng Chu, Rajit Gadh, "Predictive Scheduling for Electric Vehicles Considering Uncertainty of Load and User Behaviors", 2016 IEEE/PES Transmission and Distribution Conference and Exposition (T&D), Dallas, TX, USA, IEEE, July 25, 2016, 1 - 5, doi: 10.1109/TDC.2016.7520018
Un-coordinated Electric Vehicle (EV) charging can create unexpected load in local distribution grid, which may degrade the power quality and system reliability. The uncertainty of EV load, user behaviors and other baseload in distribution grid, is one of challenges that impedes optimal control for EV charging problem. Previous researches did not fully solve this problem due to lack of real-world EV charging data and proper stochastic model to describe these behaviors. In this paper, we propose a new predictive EV scheduling algorithm (PESA) inspired by Model Predictive Control (MPC), which includes a dynamic load estimation module and a predictive optimization module. The user-related EV load and base load are dynamically estimated based on the historical data. At each time interval, the predictive optimization program will be computed for optimal schedules given the estimated parameters. Only the first element from the algorithm outputs will be implemented according to MPC paradigm. Current-multiplexing function in each Electric Vehicle Supply Equipment (EVSE) is considered and accordingly a virtual load is modeled to handle the uncertainties of future EV energy demands. This system is validated by the real-world EV charging data collected on UCLA campus and the experimental results indicate that our proposed model not only reduces load variation up to 40% but also maintains a high level of robustness. Finally, IEC 61850 standard is utilized to standardize the data models involved, which brings significance to more reliable and large-scale implementation.
Bin Wang, Yubo Wang, Charlie Qiu, Chi-Cheng Chu, Rajit Gadh, "Event-based Electric Vehicle Scheduling Considering Random User Behaviors", 2015 IEEE International Conference on Smart Grid Communications (SmartGridComm), Miami, FL, USA, IEEE, March 21, 2016, 313-318, doi: 10.1109/SmartGridComm.2015.7436319
Uncontrolled Electric Vehicle (EV) and Plug-in Hybrid Electric Vehicle (PHEV) charging within a local distribution grid may cause unexpected high load, which further results in power quality degradation. However, coordinating charging behaviors of a number of EVs is a challenging task, which involves not only the deterministic schedule computing but also nondeterministic EV driver behaviors with random arrival time and energy demands. Previous researches in this area rarely consider these random behaviors for real EV users. In this paper, an implementable event-based cost optimal scheduling algorithm (ECSA) is developed, which solves EV scheduling problem by dynamically estimating the stay duration and energy demand for each participating EV user. Datasets, including users' historical charging records and time series meter data collected from Electric Vehicle Supply Equipments (EVSEs) in UCLA campus, are utilized for feature extraction. Based on that, proper inference technique is employed to determine parameters within each charging session. In addition, solar generation integration into EVSEs is also considered in our problem formulation. The proposed approaches are tested and validated by real EV charging schedules of users in UCLA campus. The results from simulation experiment demonstrate that the proposed algorithm has a better performance in cost minimization and load shifting compared to existing equal-sharing scheduling algorithm (ESSA).
Bin Wang, Boyang Hu, Charlie Qiu, Peter Chu, Rajit Gadh, "EV charging algorithm implementation with user price preference", 2015 IEEE Power & Energy Society Innovative Smart Grid Technologies Conference (ISGT), Washington, DC, USA, IEEE, June 25, 2015, 1 - 5, doi: 10.1109/ISGT.2015.7131895
In this paper, we propose and implement a smart Electric Vehicle (EV) charging algorithm to control the EV charging infrastructures according to users' price preferences. Charging boxes, equipped with bi-directional communication devices and smart meters, can be remotely monitored by the proposed charging algorithm applied to EV control center and mobile app. On the server side, ARIMA model is utilized to fit historical charging load data and perform day-ahead prediction. A pricing strategy with energy bidding policy is proposed and implemented to generate a charging price list to be broadcasted to EV users through mobile app. On the user side, EV drivers can submit their price preferences and daily travel schedules to negotiate with Control Center to consume the expected energy and minimize charging cost simultaneously. The proposed algorithm is tested and validated through the experimental implementations in UCLA parking lots.