Machine Learning Opens New Doors in Traumatic Brain Injury Research
Collaboration Shows how ML Can Enhance Prognoses for TBI and other Complex Medical Conditions
February 12, 2024
By Kathy Kincade
Contact: cscomms@lbl.gov
In a paper published in Nature Scientific Reports, researchers from Lawrence Berkeley National Laboratory (Berkeley Lab), the University of California, San Francisco (UCSF), the Medical College of Wisconsin, and the University of California Berkeley – in conjunction with the TRACK-TBI (Transforming Research and Clinical Knowledge in Traumatic Brain Injury) collaboration – describe how machine learning methods can enhance the prognosis and understanding of traumatic brain injury (TBI).
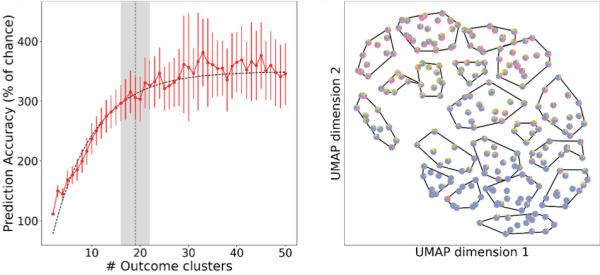
TBI patient outcomes can be predicted from patient intake data with a high degree of precision by applying a novel combination of non-parametric unsupervised and supervised machine learning. Left: Fold-over-chance accuracy of predicting outcomes into a different number of clusters. Right: Two-dimensional UMAP (Uniform Manifold Approximation and Projection) embedding clustered into 19 clusters. (Credit: Andrew Tritt and Kristofer Bouchard, Lawrence Berkeley National Laboratory)
“Our goal with this collaborative project was to bring together clinical experts in TBI who have complex, high-dimensional datasets with data scientists who have expertise in data-driven discovery,” said corresponding-author Kris Bouchard, who leads the Computing Biosciences Group in Berkeley Lab’s Scientific Data Division. “We were able to develop and apply new machine learning methods to address outstanding challenges found broadly across the entire medical community, not just TBI.”
Specifically, the team addressed two major challenges in precision medicine and personalized treatments: how to glean clinically relevant understanding from complex biomedical datasets and how to leverage that data to improve the precision of patient prognosis (prediction of patient prediction outcomes). Extracting clinically interpretable insights from large, complex datasets such as those found in TBI – which affects ~4 million people in the U.S. annually and costs the world economy $400 billion a year – is challenging and can impede prognosis and treatment.
“Current medical TBI classification frameworks typically group patients into a small number of blunt classes according to their presenting injury severity – mild, moderate, or severe – rather than looking at their individual biology and associated conditions,” said Geoffrey Manley, Professor of Neurosurgery at UCSF and Contact PI of theTRACK-TBI Pilot study. “We’ve long known, and now have the data to prove, that injury characterized as ‘mild’ in the acute post-injury timeframe can have long-term, potentially life-altering consequence and disabling chronicity [being persistent or long-lasting].”
These groupings of symptoms, termed “outcome phenotypes” by the researchers, were among the most important findings of the current analysis, he added. “Thus there is a need to find new ways to more precisely describe patients and their projected outcomes early on and to predict those longer term outcomes from the patients’ intake variables from available datasets so that we can intervene with appropriate and, in time, even preventive therapy,” stressed Manley.
“A critical first step toward personalized treatments [in TBI and other complex medical conditions] is to increase the precision with which we predict patient outcomes,” the research team noted.
Blazing New Trails
For more than a decade, the TRACK-TBI consortium has been blazing trails in the study of TBI, including carrying out the TRACK-TBI Pilot study, an important research project begun in 2017 that initially yielded a dataset comprising more than 500 intake variables collected from nearly 600 TBI patients at multiple U.S. trauma centers. Working with this dataset, the Nature Scientific Reports paper authors developed and applied interpretable machine learning techniques to the TRACK-TBI Pilot dataset across hundreds of intake variables, including socioeconomics, demographics, biomarkers, and medical data collected during initial presentation.
Their goal was to test two hypotheses: that hidden within this complex dataset were a small number of clinical concepts that described individual patients’ intake characteristics and outcome phenotypes; and that there is an unappreciated level of precision with which TBI outcome phenotypes can be predicted from those intake features.
“One thing that makes this dataset unique in both medical and biological datasets is the richness and diversity with which the individual subjects were characterized,” Bouchard said. “This allows you to identify patterns and characteristics that you may not have thought were relevant in the first place, and then provide decision support for patient prognosis.”
Using the TRACK-TBI Pilot Study dataset and machine learning capabilities developed by data scientists in Berkeley Lab’s Computing Sciences Area, the team analyzed hundreds of simulations on the Cori supercomputer at the National Energy Research Scientific Computing Center (NERSC) and found that 19 types of outcomes can be predicted from intake data – a more than six-fold improvement in precision over current clinical standards (currently limited to mild, moderate, and severe TBI), they noted. At the individual level, they found that 36% of the total outcome variance across patients can be anticipated or predicted – the first time such a prediction problem has been attempted in TBI. They also found that complex data describing TBI patients' intake characteristics and post-injury outcome phenotypes can be simplified into smaller groups of factors that doctors can easily understand, providing a complete and measurable description of each individual patient.
Interestingly, the study also points to a relationship between a patient’s socioeconomic environment and their injury that might help explain the diversity of their outcomes, Bouchard noted. “We found that one of the primary environmental modulators is their socioeconomic background. This became a differentiating factor of their intake characteristics that was important for predicting various outcome trajectories,” he said.
A Unique Combination
The machine learning approaches the collaborators applied to achieve these results represent a “first of its kind” in the study of TBI: a new interpretable machine learning algorithm known as UoI-NMF and a combination of supervised and unsupervised machine learning methods.
“We developed and applied two key algorithms for this project,” Bouchard explained. “The first was a new algorithm for Non-negative Matrix Factorization (NMF), which we used to distill the complex data into holistic factors; the second was a nonparametric machine learning approach for determining how many outcome categories could be predicted from the intake variables.”
Understanding how many types of patient outcomes, or phenotypes, exist is a central problem in TBI research. To address this problem, the team performed unsupervised learning to cluster patient outcomes and used the supervised learning method as a guide for the unsupervised process, added Andrew Tritt, a data engineer in Berkeley Lab’s Applied Mathematics and Computational Research Division and lead author on the Nature Scientific Reports paper. This enabled them to address some challenges in “clustering,” a common statistical method used to identify similar groups of data in a large dataset. Clustering uses an unsupervised learning algorithm to organize variables into groups based on how closely associated they are. The problem is, you don’t know how many clusters exist in the data before running the model.
“Clustering is where you have a dataset and you want to determine how many different things are in it, but how do you know?” Tritt said. “When you don’t have ground truth, how do you know when you’ve determined the right number?”
When a clustering algorithm is run on a dataset, the goal is to divide the dataset into a number of groups, “and there’s some stochasticity [randomness] to that process when you try to score and verify the number of clusters you find,” Tritt added. To address these and other random effects that can occur in cluster analysis, the team reran the process hundreds of times on the Cori supercomputer and then looked at the average of the results to identify the most stable groups and perform uncertainty quantification.
“That’s what makes our approach unique,” he said. “The supervised method was used to verify or score the results of the unsupervised method, where you don’t usually have a rigorous way of verifying the results. I haven’t seen that done before.” There are many cases of disparate complex datasets collected for the same biological entities, where quantifying the relationship between such datasets remains elusive, he added. “For example, studying the relationship between environmental microbiome data and ecosystem or atmospheric data. In these situations, I think the methods developed as part of this project are directly applicable for analyzing these relationships.”
In addition to Bouchard, Manley, and Tritt, co-authors on the Nature Scientific Reports paper include John Yue of Zuckerberg San Francisco General Hospital and Trauma Center and UCSF; Adam Ferguson of Zuckerberg San Francisco General Hospital and Trauma Center, UCSF, and the San Francisco Veterans Affairs Healthcare System; Abel Torres Espin of the University of Waterloo (Canada); Esther Yuh and Amy Markowitz of Zuckerberg San Francisco General Hospital and Trauma Center and UCSF; Lindsay Nelson of Medical College of Wisconsin; and the TRACK-TBI Investigators.
NERSC is a U.S. Department of Energy Office of Science user facility located at Berkeley Lab.
About Berkeley Lab
Founded in 1931 on the belief that the biggest scientific challenges are best addressed by teams, Lawrence Berkeley National Laboratory and its scientists have been recognized with 16 Nobel Prizes. Today, Berkeley Lab researchers develop sustainable energy and environmental solutions, create useful new materials, advance the frontiers of computing, and probe the mysteries of life, matter, and the universe. Scientists from around the world rely on the Lab’s facilities for their own discovery science. Berkeley Lab is a multiprogram national laboratory, managed by the University of California for the U.S. Department of Energy’s Office of Science.
DOE’s Office of Science is the single largest supporter of basic research in the physical sciences in the United States, and is working to address some of the most pressing challenges of our time. For more information, please visit energy.gov/science.