CRIC Database Brings Machine Learning to Women's Health
CRD’s Dani Ushizima is part of a team developing a searchable image database to enhance Pap smear tests
August 2, 2021
Contact: cscomms@lbl.gov
Daniela Ushizima — a staff scientist in the Computational Research Division at Lawrence Berkeley National Laboratory — and the Center for Recognition and Inspection of Cells (CRIC) have taken a major step toward applying machine learning techniques to women’s health: their newly published database of images of cervical-vaginal cytology, the CRIC Cervix Collection, offers innovative opportunities for the use of machine learning for biomedical purposes.
Their work is described in a paper published June 10 in Nature Scientific Data.
According to the World Health Organization, cervical cancer is the fourth-most common cancer in women and the leading cause of cancer death in 42 countries. Wide adoption of the cytopathological procedure known as the Pap smear has reduced incidence and death associated with cervical cancer by 65% in the past 40 years, but most Pap smears are inspected visually by humans, which can be both labor-intensive and subjective.
As a result, efforts to automate the process have increased. While machine learning has been highlighted as a way to reduce the limitations of the Pap test, the absence of high-quality curated datasets has prevented the development of strategies to improve cervical cancer screening.
The CRIC Cervix Collection is a promising start toward overcoming this challenge: at 11,534 cell images, it is the world’s largest collection of images of cervical cells collected conventionally through Pap smears. It is open source and searchable, with the aim of advancing reproducible research and FAIR (Findable, Accessible, Interoperable, and Reusable) data. Each cell image in the database was manually classified by a team of cytopathologists using the Bethesda System, a standardized nomenclature for cervicovaginal cytology.
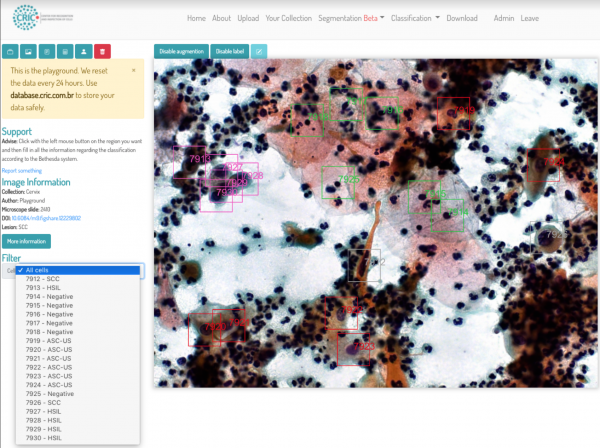
This screenshot from the CRIC database shows several HSIL (high grade squamous intraepithelial lesions), which are lesions of a pre-cancerous disease.
This manual portion of the process is what makes future machine-learning achievements in this area possible: before algorithms for cell sample analysis can be developed, the database must be seeded with a broad range of images, each of which must come with consistent, standardized metadata.
“We had this chicken-egg situation where we wanted to design a program to help us with detection, but in order to do so we needed standards to verify if the code was accurate,” said Ushizima, a co-founder of CRIC and a research fellow in the Berkeley Institute for Data Science. “And in order to make sure the code was accurate, standards were required. And the community didn’t have good standards for this task. But now we do.”
With this work done, scientists began using the data and designing algorithms to accurately screen for cervical cancer — and using the platform itself as a model for other work. As an example, an overview of a deep learning ensemble method was recently published in the Journal of Imaging 2021, where the researchers discussed the use of this database as a reference for more accurate cell classification (“A deep learning ensemble method to assist cytopathologists in Pap test image classification”).
“One can use the platform to search for curated data and use the data in one way or the other by downloading all these datasets — they’re free for download, including all the metadata — but also, new collections can be uploaded,” said Ushizima. “So it’s not just a CRIC Cervix data research tool; it’s a cell data research tool that allows users to handle different collections.”
In the immediate future, the CRIC Cervix platform may also serve as a model for teams studying neurons and samples of pleural effusions, another type of sample that currently requires manual intervention by a human cytopathologist.
Why does this type of database lend itself so well to so many machine-learning applications? It’s all about the mathematics of visual analysis.
“One of the initiatives I work with on the UC Berkeley campus, Images Across Domains, brings together people working with images of rocks, images of biofuel, images of batteries, images of cancer, and asking is there anything these have in common?” said Ushizima. “The answer is ‘yes.’ The common language is in the mathematics behind the algorithms that allow us to detect objects or structure and recognize the different properties associated with the color of the pictures, with texture, and with shape. That mathematics is the mathematics of matrix calculations.”
That common language offers broad opportunities for advancing the development and adoption of machine learning techniques across a number of scientific disciplines, as other teams and other disciplines create similar platforms. And with time, the use of machine learning in the CRIC Cervix Collection and other contexts could very well save lives around the world.
About Berkeley Lab
Founded in 1931 on the belief that the biggest scientific challenges are best addressed by teams, Lawrence Berkeley National Laboratory and its scientists have been recognized with 16 Nobel Prizes. Today, Berkeley Lab researchers develop sustainable energy and environmental solutions, create useful new materials, advance the frontiers of computing, and probe the mysteries of life, matter, and the universe. Scientists from around the world rely on the Lab’s facilities for their own discovery science. Berkeley Lab is a multiprogram national laboratory, managed by the University of California for the U.S. Department of Energy’s Office of Science.
DOE’s Office of Science is the single largest supporter of basic research in the physical sciences in the United States, and is working to address some of the most pressing challenges of our time. For more information, please visit energy.gov/science.