Zhe Bai
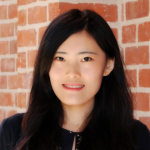
Zhe
(Eliza)
Bai
Computational science researcher
Computing Sciences Area
Phone: +1 (510) 486-4294
1 Cyclotron Road
M/S 59R3103
Berkeley,
CA
94720
Zhe Bai is a computational science researcher in the Computing Sciences Area at Lawrence Berkeley National Laboratory. Her research interests lie in the area of sparse sampling and model order reduction, including compressed sensing, machine learning and large-scale computation and simulation. Cultivated interdisciplinary research and collaborations spanning the fields of engineering and applied mathematics, her work involves data-driven modeling that leverages advanced data science techniques to understand, estimate and control high-dimensional physical systems.
Education
- Ph.D., Mechanical Engineering, University of Washington, 2018.
- M.S., Applied Mathematics, University of Washington, 2017.
- M.S., Mechanical & Aerospace Engineering, Syracuse University, 2013.
- B.S., Thermal Energy & Power Engineering, Harbin Institute of Technology, 2011.
Previous Appointments
- Research Assistant, University of Washington, Seattle, WA.
- Research Intern, Sandia National Laboratories, Livermore, CA.
- Research Assistant, Syracuse University, Syracuse, NY.