Rafael Zamora-Resendiz
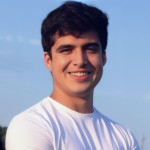
Rafael Zamora-Resendiz is a Computer Systems Engineer (CSE-2) in the Applied Mathematics and Computational Research Division (AMCR) at Lawrence Berkeley National Laboratory (LBNL). Currently, his focus is on applied Natural Language Processing (NLP) for healthcare, collaborating closely with the Department of Veterans Affairs (VA) as part of the Million Veterans Program (MVP). In this role, he spearheads the development of NLP methods for scalable information retrieval, utilizing high-performance computing.
Rafael earned his Bachelor of Science degree in Computer Science from Hood College in 2017, gaining practical experience conducting scientific research during his Visiting Faculty Program (VFP) internship under the guidance of Dr. Xinlian Liu (Hood College) and Dr. Silvia Crivelli (LBNL). His postbaccalaureate research explored applications of deep learning to structural proteomics, developing methods for representing structural information in machine learning models.
Joining the Applied Computing for Scientific Discovery (ACSD) group as a domain expert in machine learning in 2019, Rafael provides methodological support to VA clinicians in developing and implementing deep learning-enabled electronic health record analysis. His efforts include the development of large language models for clinical text and the creation of scalable search algorithms, specifically aimed at indexing U.S. Veteran mortality factors.
Rafael recently secured an INCITE Award for FY2024 under the project titled "Clinical Foundation LLMs for Scaling Public Health Surveillance." Serving as a Co-Investigator under Dr. Silvia Crivelli, this project will harnesses the computational capabilities of Oak Ridge National Laboratory's HPE-Cray EX, with an allocation of 200,000 Frontier node-hours. The research will contribute to advancing biomedical science by leveraging large language models (LLMs) for precision healthcare. In developing a foundational model for clinical language using the Veteran Affair’s Corporate Data Warehouse (CDW), the team aims to expand the size of clinical LLMs by tens of billions in parameters and train them on a corpus comprising billions of documents, drawn from over 20 years of clinical text for over 23 million patients.
Rafael's goal is to leverage emerging foundation models towards building a comprehensive "map of disease progression" for the U.S. veteran population, which will help in understanding the applicability of pre-trained LLMs for representing clinically meaningful phenotypes and monitoring impacts of medical interventions on patient health over time.