Berkeley Lab Researchers Help Accelerate Fusion Progress Through Machine Learning Algorithms
April 1, 2025
By Owen Poindexter
Contact: cscomms@lbl.gov
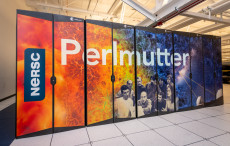
Researchers at Berkeley Lab participated in a collaboration with other institutions such as Princeton Plasma Physics Laboratory, MIT Plasma Science Fusion Center, San Francisco State University and CEA-IRFM to generate machine learning models that accelerated predictions of plasma heating in fusion energy devices. The team used NERSC’s Cori and Perlmutter supercomputers to train the ML-models. (Credit: Elizabeth Ball)
A significant advance in the use of machine learning (ML) algorithms could accelerate fusion energy research. Previous research at the Massachusetts Institute of Technology (MIT) has shown how physicists can utilize machine learning techniques to conduct their research, such as tracking plasma turbulence during fusion experiments. Now, researchers at Lawrence Berkeley National Laboratory (Berkeley Lab) have collaborated with the Princeton Plasma Physics Laboratory (PPPL), MIT’s Plasma Science and Fusion Center, San Francisco State University (SFSU), and French Alternatives Energies and Atomic Energy Commission (CEA-IRFM), to develop machine learning (ML) models that accelerate predictions of particle heating in fusion plasmas via radiofrequency (RF) waves. The speed of these models will allow the researchers to predict how the waves heat up the plasma not only between tests, but also in real-time so that they can make adjustments during the operation of the device. Ultimately, this research could catalyze fusion energy research.
Nuclear fusion, the process that powers the sun, occurs when atomic nuclei collide and fuse, releasing a significant amount of energy. In laboratory conditions, this requires heating a plasma of hydrogen isotopes to temperatures exceeding 100 million degrees Celsius inside devices such the donut-shaped reactors called tokamaks, which use powerful magnetic fields to confine the plasma. Harnessing that energy on Earth involves reaching these extreme temperatures, containing the reaction magnetically, and ultimately producing more energy than the system consumes.
“Plasma dynamics are driven by complex phenomena across a diverse range of timescales from microseconds to minutes,” explains Álvaro Sánchez Villar, the Associate Research Physicist at PPPL who led this research. Traditional computational simulations have allowed insights into these physical processes but have also had computational costs restricting their use in real-time decision-making. Real-time fusion control systems require operations on the order of milliseconds, while simulating the ion cyclotron range of frequencies (ICRF) heating in tokamaks can take minutes. Machine learning models can help speed up the predictions of these processes. Sánchez Villar’s team wanted to generate models that would predict plasma heating, resulting in real-time control.
Talita Perciano and Zhe Bai, researchers at Berkeley Lab, helped Sánchez Villar’s team and other labs develop ML algorithms to accelerate radiofrequency heating simulations. “These models predict heating six orders of magnitude faster than the original model, while also preserving accuracy” explained Sánchez Villar.
The resulting models were designed to predict the heating in two different fusion devices, the National Spherical Torus Experiment-Upgrade (NSTX‑U) based at the Princeton Plasma Physics Laboratory and the Tungsten (W) Environment in Steady-state Tokamak (WEST) located in Saint-Paul-lès-Durance in southern France. Their collaborator from CEA-IRFM contributed by providing the expertise and data critical to generate the database for WEST.
“Solving the computational bottleneck opens possibilities for more complex and numerous simulations,” explains Perciano, Principal Investigator at Berkeley Lab. "This could eventually enable real-time adjustments during actual tokamak operations."
“We employ efficient sampling techniques and multiple machine learning models to optimize the computational performance during inference,” added Bai, a developer at LBNL. “This work contributes to the design and real-time control of tokamak-based fusion power plants.”
Sánchez Villar built upon the methods used at Berkeley Lab and consulted with the LBNL team throughout the process. “The team at Berkeley Lab helped me with the project startup,” Sánchez Villar explained, “understanding algorithms, and helped familiarize myself with the methods that provided guidance when developing the models.” His team used two supercomputers at the National Energy Research Scientific Computing Center (NERSC), Cori and Perlmutter, to run simulations for the database. “We ran the simulations for the database in Cori,” says Sánchez Villar, “the most computationally intensive tasks were the surrogate model training and hyperparameter optimization, which were carried out on Perlmutter.”
The research, published in the journal Nuclear Fusion, focused on modeling electron and ion behavior under a plasma RF-heating method known as ion cyclotron range of frequencies (ICRF), in which RF-waves are launched to heat the plasma particles. The team's ML algorithms demonstrated remarkable improvements in processing speed compared to traditional simulation methods, while maintaining high accuracy in predicting plasma heating behavior. Looking ahead, Perciano highlights the broader implications of the work: "These methods and algorithms are highly flexible and can be adapted to advance a wide variety of challenges in fusion energy research.”
These computational advancements come at a critical time in fusion research, as laboratories and private companies work toward achieving sustainable fusion power. The ability to quickly simulate and predict plasma behavior could accelerate progress across the field, bringing us closer to a future of abundant, clean energy. The research team's work demonstrates how advanced computing and ML can overcome long-standing challenges in fusion research, potentially shortening the timeline to practical fusion energy. As the international fusion research community continues to advance, these computational tools will play an increasingly important role in designing and optimizing future fusion reactors.
This work was supported by the U.S. Department of Energy under Contract Number DE-AC02-09CH1146 and Contract Number DE-SC0021202. This research used resources of the National Energy Research Scientific Computing Center (NERSC), a U.S. Department of Energy Office of Science User Facility located at Lawrence Berkeley National Laboratory, operated under Contract No. DE-AC02-05CH11231 using NERSC award FES m3716 for 2023.
About Berkeley Lab
Founded in 1931 on the belief that the biggest scientific challenges are best addressed by teams, Lawrence Berkeley National Laboratory and its scientists have been recognized with 16 Nobel Prizes. Today, Berkeley Lab researchers develop sustainable energy and environmental solutions, create useful new materials, advance the frontiers of computing, and probe the mysteries of life, matter, and the universe. Scientists from around the world rely on the Lab’s facilities for their own discovery science. Berkeley Lab is a multiprogram national laboratory, managed by the University of California for the U.S. Department of Energy’s Office of Science.
DOE’s Office of Science is the single largest supporter of basic research in the physical sciences in the United States, and is working to address some of the most pressing challenges of our time. For more information, please visit energy.gov/science.