Unraveling Brain Complexity
New AI Method Aids Complex Data Interpretation
May 22, 2024
By Carol Pott
Contact: cscomms@lbl.gov
Within the intricate workings of the brain, groups of neurons coordinate, enabling our ability to hear sounds, perform intricate movements of our limbs, and delve into complex thoughts and plans. Understanding if and how things in the outside world, like the pitch of a melody or the angle of an arm movement, influence the collective actions of these neuron clusters is fundamental to understanding how the brain works and unraveling its mysteries. Complex brain activity can be simplified into discernible patterns that showcase how groups of neurons are activating over time.
However, current analysis techniques often fail to reveal the direct connections between these patterns and external factors like sounds or movements. This failure has sparked a lively debate about our perception and comprehension of the brain: is it a dynamical system orchestrating perception, cognition, and action, or a sophisticated computing engine directly manipulating sensory, cognitive, and behavioral data?
New research published in PLOS Computational Biology by a team in Lawrence Berkeley National Laboratory’s (Berkeley Lab’s) Scientific Data (SciData) Division and the Biological Systems and Engineering Division reveals a fascinating truth: the brain operates as both. The brain orchestrates perception and action through the synchronized dynamics of populations of neurons, each meticulously organized to mirror the world around us. This detailed organization enables sophisticated computations on these representations, supporting advanced mental functions related to perception and action.
The team achieved this finding by developing a novel, interpretable, unsupervised AI model and applying it to brain activity data. Called the orthogonal stochastic linear mixing model (OSLMM), a type of Gaussian process, the tool allows researchers to understand how groups of neurons work together over time. A Gaussian process is a powerful tool that models intricate observations by breaking them down into simpler functions. It not only measures the uncertainty surrounding these functions but also empowers predictions and inference, making it a versatile asset in data analysis. While Gaussian processes are commonly used for prediction and uncertainty quantification, the team used OSLMM to inspect those simple functions to gain insight into the unknown processes that generate the observed brain data. OSLMM is a Bayesian regression framework and utilizes Markov chain Monte Carlo inference procedures, which are statistical techniques used to estimate model parameters.
The team applied OSLMM on real brain data from animals: the brain activity of rats listening to pure tones (collected in the Neural Systems and Data Science Lab at Berkeley Lab) and previously collected data from monkeys performing complex arm movements (from Sabes Lab, University of California, San Francisco). OSLMM was able to accurately predict how the neurons would act and how the animals would move. OSLMM also revealed that the underlying patterns of brain activity directly relate to the sounds and movements the animals were experiencing. Together, these results suggest that OSLMM will be useful for learning interpretable and predictive AI models for the analysis of complex time-series datasets.
"Understanding how things work in biology, like brain functions, is tough because often it is the coordination of many individual components (e.g., neurons, molecules, microbes, etc.) that produce an interesting phenomenon. There are a lot of data observations, and they are noisy. We are also unable to observe all relevant components simultaneously,” said Kris Bouchard, leader of SciData’s Computational Biosciences Group and PI of the Neural Systems and Data Science Lab in the Biological Systems and Engineering Division. “OSLMM discovers hidden patterns better and is more scalable than other methods. While we created OSLMM to address problems in neuroscience, the method is completely general and can be applied to other complex time-series data sets.”
Breaking Down the Methodologies of Research
There are many ways to understand complex time-series data. In neuroscience, one popular method is called Gaussian Process Factor Analysis (GPFA), which looks at how neurons work together over time. GPFA assumes that the ‘interactions’ between neurons stay the same over time, which isn’t always true in real brain data. Other methods, like neural networks, try to capture how the brain changes over time in more flexible ways and can maximize metrics like predictive accuracy but generally do not provide interpretable insights into the processes that generated the data. Interpretable insights are a critical need for scientific ML/AI that differs from the needs of industrial ML/AI.
Compared to GPFA, OSLMM showed better performance in recovering hidden patterns in experiments and predicting outcomes in real data. Importantly, OSLMM revealed meaningful patterns in brain recordings. For example, in rat brain recordings, OSLMM showed patterns related to sound properties, and in monkey brain recordings, it showed patterns linked to arm movements that were undetected by GPFA. These findings suggest that how the brain works may be more complex than we thought, and OSLMM helps us understand these processes better. Overall, OSLMM helped uncover hidden structures in brain data that provided insights into how the brain functions.
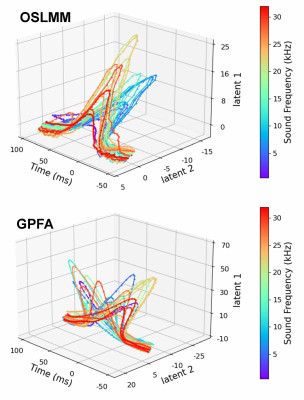
The average hidden brain activity patterns across trials for different sound frequencies, using OSLMM (top) and GPFA (bottom). The patterns discovered by OSLMM were directly organized by the sound frequency (blue-to-green-to-orange-to-red), while the patterns discovered by GPFA were not.
Simplifying Findings
OSLMM outperformed GPFA, showing an improved ability to capture meaningful patterns and predict outcomes related to external factors. OSLMM achieved these advancements through two mathematical innovations: a flexible model that allowed the interactions of neurons to change over time and imposed an orthogonality constraint on how underlying patterns relate. An orthogonality constraint refers to a restriction that ensures certain aspects or components are unrelated to each other. In this context, it means enforcing a condition where specific patterns or factors are kept separate or orthogonal from one another without influencing or overlapping with each other. The team demonstrated that this constraint significantly improves scalability, both theoretically and in practical tests across various real-world datasets. These broad modeling capabilities, along with the robust results in fields beyond neuroscience, indicate that OSLMM is a promising tool for understanding the complex systems that generate observed data.
“OSLMM has been shown to be effective for unsupervised analysis of complex data with many hidden patterns over time. It performs well in predicting outcomes, but more importantly, it revealed that the hidden patterns were organized around external factors,” said Bouchard. “This is helpful not just in neuroscience but also in other areas of biology, where we collect a lot of data from various sensors over time but are not able to measure all relevant information. The new mathematics underlying OSLMM’s ability to learn predictive and interpretable hidden patterns make it valuable for understanding complex processes and discovering new insights from large datasets.”
This research was funded by the Department of Energy Office of Science Advanced Scientific Computing Research (ASCR) AI-codesign for Complex Data program, the National Institute of Health (NINDS), and Berkeley Lab’s Laboratory Directed Research and Development.
About Berkeley Lab
Founded in 1931 on the belief that the biggest scientific challenges are best addressed by teams, Lawrence Berkeley National Laboratory and its scientists have been recognized with 16 Nobel Prizes. Today, Berkeley Lab researchers develop sustainable energy and environmental solutions, create useful new materials, advance the frontiers of computing, and probe the mysteries of life, matter, and the universe. Scientists from around the world rely on the Lab’s facilities for their own discovery science. Berkeley Lab is a multiprogram national laboratory, managed by the University of California for the U.S. Department of Energy’s Office of Science.
DOE’s Office of Science is the single largest supporter of basic research in the physical sciences in the United States, and is working to address some of the most pressing challenges of our time. For more information, please visit energy.gov/science.