Berkeley Lab Researchers Advance AI-Driven Plant Root Analysis
Enhancing Biomass Assessment and Plant Root Growth Monitoring in Hydroponic Systems
June 20, 2024
Contact: cscomms@lbl.gov
In a world striving for sustainability, understanding the hidden half of a living plant – the roots – is crucial. Roots are not just an anchor; they are a dynamic interface between the plant and soil, critical for water uptake, nutrient absorption, and, ultimately, the survival of the plant. In an investigation to boost agricultural yields and develop crops resilient to climate change, scientists from Lawrence Berkeley National Laboratory’s (Berkeley Lab’s) Applied Mathematics and Computational Research (AMCR) and Environmental Genomics and Systems Biology (EGSB) Divisions have made a significant leap. Their latest innovation, RhizoNet, harnesses the power of artificial intelligence (AI) to transform how we study plant roots, offering new insights into root behavior under various environmental conditions.
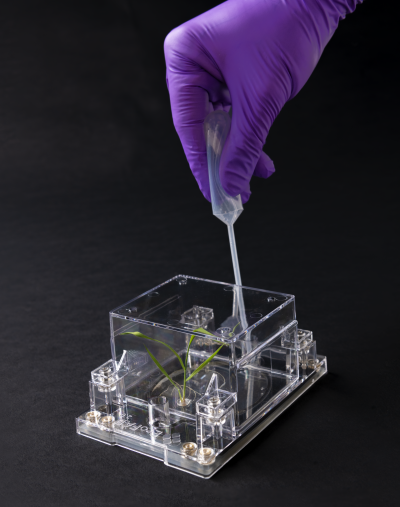
RhizoNet harnesses the power of artificial intelligence to transform how we study plant roots, offering new insights into root behavior under various environmental conditions. It uses EcoFAB (shown here), a novel hydroponic device that facilitates in-situ plant imaging by offering a detailed view of plant root systems. (Credit: Thor Swift, Berkeley Lab)
This pioneering tool, detailed in a study published on June 5 in Scientific Reports, revolutionizes root image analysis by automating the process with exceptional accuracy. Traditional methods, which are labor-intensive and prone to errors, fall short when faced with the complex and tangled nature of root systems. RhizoNet steps in with a state-of-the-art deep learning approach, enabling researchers to track root growth and biomass with precision. Using an advanced deep learning-based backbone based on a convolutional neural network, this new computational tool semantically segments plant roots for comprehensive biomass and growth assessment, changing the way laboratories can analyze plant roots and propelling efforts toward self-driving labs.
As Berkeley Lab’s Daniela Ushizima, lead investigator of the AI-driven software, explained, “The capability of RhizoNet to standardize root segmentation and phenotyping represents a substantial advancement in the systematic and accelerated analysis of thousands of images. This innovation is instrumental in our ongoing efforts to enhance the precision in capturing root growth dynamics under diverse plant conditions.”
Getting to the Roots
Root analysis has traditionally relied on flatbed scanners and manual segmentation methods, which are not only time-consuming but also susceptible to errors, particularly in extensive multi-plant studies. Root image segmentation also presents significant challenges due to natural phenomena like bubbles, droplets, reflections, and shadows. The intricate nature of root structures and the presence of noisy backgrounds further complicate the automated analysis process. These complications are particularly acute at smaller spatial scales, where fine structures are sometimes only as wide as a pixel, making manual annotation extremely challenging even for expert human annotators.
EGSB recently introduced the latest version (2.0) of EcoFAB, a novel hydroponic device that facilitates in-situ plant imaging by offering a detailed view of plant root systems. EcoFAB – developed via a collaboration between EGSB, the DOE Joint Genome Institute (JGI), and the Climate & Ecosystem Sciences division at Berkeley Lab – is part of an automated experimental system designed to perform fabricated ecosystem experiments that enhance data reproducibility. RhizoNet, which processes color scans of plants grown in EcoFAB that are subjected to specific nutritional treatments, addresses the scientific challenges of plant root analysis. It employs a sophisticated Residual U-Net architecture (an architecture used in semantic segmentation that improves upon the original U-Net by adding residual connections between input and output blocks within the same level, i.e. resolution, in both the encoder and decoder pathways) to deliver root segmentation specifically adapted for EcoFAB conditions, significantly enhancing prediction accuracy. The system also integrates a convexification procedure that serves to encapsulate identified roots from time series and helps quickly delineate the primary root components from complex backgrounds. This integration is key for accurately monitoring root biomass and growth over time, especially in plants grown under varied nutritional treatments in EcoFABs.
To illustrate this, the new Scientific Reports paper details how the researchers used EcoFAB and RhizoNet to process root scans of Brachypodium distachyon (a small grass species) plants subjected to different nutrient deprivation conditions over approximately five weeks. These images, taken every three to seven days, provide vital data that help scientists understand how roots adapt to varying environments. The high-throughput nature of EcoBOT, the new image acquisition system for EcoFABs, offers research teams the potential for systematic experimental monitoring – as long as data is analyzed promptly.
“We’ve made a lot of progress in reducing the manual work involved in plant cultivation experiments with the EcoBOT, and now RhizoNet is reducing the manual work involved in analyzing the data generated,” noted Peter Andeer, a research scientist in EGSB and a lead developer of EcoBOT, who collaborated with Ushizima on this work. “This increases our throughput and moves us toward the goal of self-driving labs.” Resources at the National Energy Research Scientific Computing Center (NERSC) – a U.S. Department of Energy (DOE) user facility located at Berkeley Lab – were used to train RhizoNet and perform inference, bringing this capability of computer vision to the EcoBOT, Ushizima noted.
“EcoBOT is capable of collecting images automatically, but it was unable to determine how a plant responds to different environmental changes,” Ushizima explained. “By measuring the roots with RhizoNet, we capture detailed data on root biomass and growth not solely to determine plant vitality but rather to provide comprehensive, quantitative insights that are not readily observable through conventional means. After training the model, it can be reused for multiple experiments (unseen plants).”
“In order to analyze the complex plant images from the EcoBOT, we created a new convolutional neural network for semantic segmentation," added Zineb Sordo, a computer systems engineer in AMCR working as a data scientist on the project. "Our goal was to design an optimized pipeline that uses prior information about the time series to improve the model's accuracy beyond manual annotations done on a single frame. RhizoNet handles noisy images, detecting plant roots from images so biomass and growth can be calculated.”
One Patch at a Time
During model tuning, the findings indicated that using smaller image patches significantly enhances the model's performance. In these patches, each neuron in the early layers of the artificial neural network has a smaller receptive field. This allows the model to capture fine details more effectively, enriching the latent space with diverse feature vectors. This approach not only improves the model's ability to generalize to unseen EcoFAB images but also increases its robustness, enabling it to focus on thin objects and capture intricate patterns despite various visual artifacts.
Smaller patches also help prevent class imbalance by excluding sparsely labeled patches – those with less than 20% of annotated pixels, predominantly background. The team’s results show high accuracy, precision, recall, and Intersection over Union (IoU) for smaller patch sizes, demonstrating the model's improved ability to distinguish roots from other objects or artifacts.
To validate the performance of root predictions, the paper compares predicted root biomass to actual measurements. Linear regression analysis revealed a significant correlation, underscoring the precision of automated segmentation over manual annotations, which often struggle to distinguish thin root pixels from similar-looking noise. This comparison highlights the challenge human annotators face and showcases the advanced capabilities of the RhizoNet models, particularly when trained on smaller patch sizes.
This study demonstrates the practical applications of RhizoNet in current research settings, the authors noted, and lays the groundwork for future innovations in sustainable energy solutions as well as carbon-sequestration technology using plants and microbes. The research team is optimistic about the implications of their findings.
“Our next steps involve refining RhizoNet’s capabilities to further improve the detection and branching patterns of plant roots,” said Ushizima. "We also see potential in adapting and applying these deep-learning algorithms for roots in soil as well as new materials science investigations. We're exploring iterative training protocols, hyperparameter optimization, and leveraging multiple GPUs. These computational tools are designed to assist science teams in analyzing diverse experiments captured as images, and have applicability in multiple areas.”
Further research work in plant root growth dynamics is described in a pioneering book on autonomous experimentation edited by Ushizima and Berkeley Lab colleague Marcus Noack that was released in 2023. Other team members from Berkeley Lab include Peter Andeer, Trent Northen, Camille Catoulos, and James Sethian. This multidisciplinary group of scientists is part of Twin Ecosystems, a DOE Office of Science Genomic Science Program project that integrates computer vision software and autonomous experimental design software developed at Berkeley Lab (gpCAM) with an automated experimental system (EcoFAB and EcoBOT) to perform fabricated ecosystem experiments and enhance data reproducibility. The work of analyzing plant roots under different kinds of nutrition and environmental conditions is also part of the DOE’s Carbon Negative Earthshot initiative (see sidebar).
Related Reading:
Berkeley Lab Awarded Two New Centers to Counter Climate Change
Using Deep Learning to Better Assess Lithium Metal Battery Performance
Berkeley Lab Researchers Publish Pioneering Book on Autonomous Experimentation
EcoFAB 2.0 devices are being used in multiple outreach activities
Recognition Software Drives Matches across Multiple Science Domains
About Berkeley Lab
Founded in 1931 on the belief that the biggest scientific challenges are best addressed by teams, Lawrence Berkeley National Laboratory and its scientists have been recognized with 16 Nobel Prizes. Today, Berkeley Lab researchers develop sustainable energy and environmental solutions, create useful new materials, advance the frontiers of computing, and probe the mysteries of life, matter, and the universe. Scientists from around the world rely on the Lab’s facilities for their own discovery science. Berkeley Lab is a multiprogram national laboratory, managed by the University of California for the U.S. Department of Energy’s Office of Science.
DOE’s Office of Science is the single largest supporter of basic research in the physical sciences in the United States, and is working to address some of the most pressing challenges of our time. For more information, please visit energy.gov/science.