Advancing New Battery Design with Deep Learning
AMCRD’s Dani Ushizima Outlines New Lithium Metal Battery Research at APS Meeting
April 4, 2022
By Keri Troutman
Contact: cscomms@lbl.gov
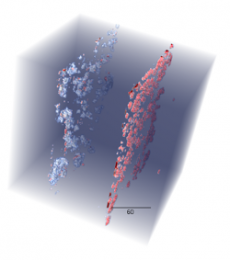
Semantic segmentation: Automated detection of dendrites (blue) and pitts (red) using Y-net, a deep-learning algorithm developed to automate the quality control and assessment of new battery designs that was run at NERSC on the Cori and Perlmutter systems.
Electric cars are an integral part of our clean energy future – every time one replaces a gas-powered vehicle, it can save 1.5 tons of carbon dioxide per year. But to truly expand the population and reach of electric cars, new energy storage solutions must be developed to produce lighter vehicles with longer ranges and more powerful batteries.
A team of researchers from Lawrence Berkeley National Laboratory (Berkeley Lab) and UC Irvine recently moved this effort forward with the development of deep-learning algorithms to automate the quality control and assessment of new battery designs.
The research team, led by Berkeley Lab’s Daniela Ushizima, a staff scientist in the Applied Mathematics and Computational Research Division and a Berkeley Institute for Data Science Research Affiliate, includes scientists from the National Fuel Cell Research Center (NFRC) at UC Irvine and collaborators from UC Berkeley’s Department of Electrical Engineering and Computer Sciences and School of Information. Together, they created these deep-learning algorithms to automate the inspection of batteries with data acquired using advanced instruments, including those at Berkeley Lab’s Advanced Light Source (ALS). By using X-ray tomography as the input data, as well as prototypes defined by battery experts, the research team developed automated methods to detect battery defects in rechargeable lithium metal batteries and measure their growth during battery cycling.
The researchers focused on solid-state lithium metal batteries (LMB), which are different from traditional lithium-ion batteries in that they use solid electrodes and electrolytes, providing superior electrochemical performance and high energy density.
“This means increased charge storage capacity and the ability to hold almost twice the energy of a traditional lithium ion battery – higher energy density at lower battery weight and size,” said Ushizima, who provided more details about this research during a presentation, "Lithium Metal Battery Characterization using X-ray Imaging and Machine Learning," at the American Physical Society Meeting on March 16. Her co-authors included Jerome Quenum, David Perlmutter, and Dula Parkinson of Berkeley Lab and Ying Huang and Iryna Zenyuk of the NFCR.
Some of the challenges of this new technology are predicting battery cycling stability and preventing the formation of lithium dendrite growth, Ushizima noted. This harmful phenomenon may occur during LMB charge and discharge, when lithium can deposit irregularly, building up dendrites (lithium plating) that lead to failures, such as short-circuiting. These morphologies are key to the LMB quality, and they can be captured and analyzed using X-ray microtomography (XRT) scans. Machine learning algorithms and multiscale representation of XRT from LMB samples enable the quantification of LMB defects.
“Quality control and quality assessment require quantifiable metrics, which means detecting and measuring dendrites automatically,” Ushizima explained. To do this requires running semantic segmentation by, for example, using convolutional neural networks (CNNs). Among the variety of segmentation algorithms based on CNN, one of the first CNNs the research team tested was a U-net scalable implementation they recently published in Nature Scientific Data.
“The advantage of using our U-net was the ability to quickly adapt it from ceramic matrix composite images to the new microCT data coming from batteries,” she said. “Our latest efforts are centered on state-of-the-art algorithms such as vision transformers. One of the advantages is the ability to learn a sequence of representations from the microCT data as part of capturing the multiscale aspects of microCT microstructures.”
“That’s what we do: We create deep-learning algorithms to automate the inspection of battery data acquired with advanced imaging instruments at U.S. Department of Energy (DOE) national laboratories, including Berkeley Lab’s ALS and Argonne’s Advanced Photon Source (APS) facilities,” added Ushizima, who is also affiliated with Berkeley Lab’s Energy Storage Center
The ALS and APS are DOE Office of Science user facilities.
About Berkeley Lab
Founded in 1931 on the belief that the biggest scientific challenges are best addressed by teams, Lawrence Berkeley National Laboratory and its scientists have been recognized with 16 Nobel Prizes. Today, Berkeley Lab researchers develop sustainable energy and environmental solutions, create useful new materials, advance the frontiers of computing, and probe the mysteries of life, matter, and the universe. Scientists from around the world rely on the Lab’s facilities for their own discovery science. Berkeley Lab is a multiprogram national laboratory, managed by the University of California for the U.S. Department of Energy’s Office of Science.
DOE’s Office of Science is the single largest supporter of basic research in the physical sciences in the United States, and is working to address some of the most pressing challenges of our time. For more information, please visit energy.gov/science.