Machine Learning Workshop
CRD Workshop Brings Together Science Challenges and Potential Machine Learning Solutions
November 8, 2013
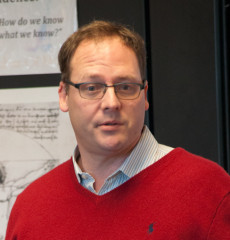
Peter Nugent, leader of CRD’s Computational Cosmology Center and the Data Analytics Team at NERSC, delivered the keynote talk on “Machine Learning in Astrophysical Surveys.”

CRD's Taghrid Samak helped organize the workshop.
As part of the Computational Research Division’s strategic emphasis on increasing the role of computation in all aspects of scientific discovery, CRD staff organized a one-day workshop on Machine Learning for Science. The meeting was held Nov. 4 at Berkeley Lab.
In many areas of science, the generation of data is now often outstripping the abilities of researchers to manage, analyze and understand the data. Machine learning, the development and use of advanced techniques to automatically classify data, detect patterns or extract results, is arguably the most widely used methodology to deal with data of this size and complexity.
The workshop, which drew more than 70 participants, specifically looked at increasing the role of machine learning in the areas of climate research, cosmology, materials, microtomography and metagenomics. About one-third of the participants were machine learning experts from universities, including UC Berkeley, UC Davis, Stanford and Rensselaer Polytechnic Institute. The others were mainly domain scientists and computer scientists From Berkeley Lab, the Stanford Linear Accelerator Center and the Department of Energy’s Joint Genome Institute (JGI).
“The idea was to get people in the same room and get them talking about the kinds of science challenges that could be addressed by machine learning,” said Taghrid Samak of the organizing committee. “In some cases, academics with expertise in machine learning theory are looking for data sets to work with, and we have a lot of really large data sets here at LBNL. And even in those areas where large problems have been solved, there are many, many sub-problems that fall under different areas of machine learning.”
To set the stage, Peter Nugent, leader of CRD’s Computational Cosmology Center and the Data Analytics Team at NERSC, gave the keynote talk on “Machine Learning in Astrophysical Surveys.” According to Nugent, machine learning is critical to maximizing the science from several large-scale astronomy surveys now under way. Combining high-bandwidth connections with high performance computing and machine learning algorithms provides near-real time turnaround for astrophysicists studying both the static universe and transitory events like supernovae.
Other talks were
- “Extreme weather event detection and characterization, “ by Michael Wehner and Prabhat of CRD
- “Informatics approaches for materials discovery,” by Anubhav Jain of the Environmental Energy Technologies Division and David Skinner of NERSC
- “Quantification of microstructures from microtomography images,” by Dilworth Parkinson of the Advanced Light Source and Daniela Ushizima of CRD
- “Functional gene annotation for metagenomics,” by Amrita Pati of JGI and Samak of CRD.
Workshop organizers wanted to give the participants from the machine learning side a substantive understanding of the science involved so that the needs of the domain scientists were clear before new approaches were suggested. “It takes real effort to get to know the science, but once you bridge that gap the two groups can start looking at the best way to apply machine learning to the problem,” Samak said.
The participants then met in small breakout groups to look at specific science domains. In the metagenomics session, the scientists explained their science, then described their sticking points. The machine learning participants suggested a few techniques that could be implemented and assessed in the short term. They then agreed to check back with each other in a few months.
In the session on materials discovery, in which researchers can combine different combinations of materials for applications such as improved battery design, the group came up with the idea of holding a “hackathon” to go through the database of tens of thousands of chemical combinations.
CRD plans to organize follow-on meetings with each of the science domains represented at the workshop.
About Berkeley Lab
Founded in 1931 on the belief that the biggest scientific challenges are best addressed by teams, Lawrence Berkeley National Laboratory and its scientists have been recognized with 16 Nobel Prizes. Today, Berkeley Lab researchers develop sustainable energy and environmental solutions, create useful new materials, advance the frontiers of computing, and probe the mysteries of life, matter, and the universe. Scientists from around the world rely on the Lab’s facilities for their own discovery science. Berkeley Lab is a multiprogram national laboratory, managed by the University of California for the U.S. Department of Energy’s Office of Science.
DOE’s Office of Science is the single largest supporter of basic research in the physical sciences in the United States, and is working to address some of the most pressing challenges of our time. For more information, please visit energy.gov/science.