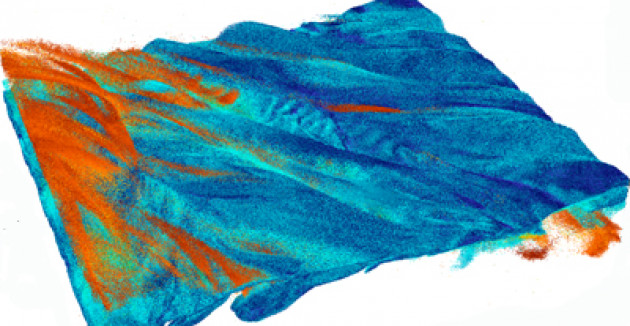
Sifting Through a Trillion Electrons
SDM's Surendra Byna and colleagues from Berkeley Lab’s Computational Research Division teamed up with researchers to develop novel software strategies for storing, mining, and analyzing massive datase…
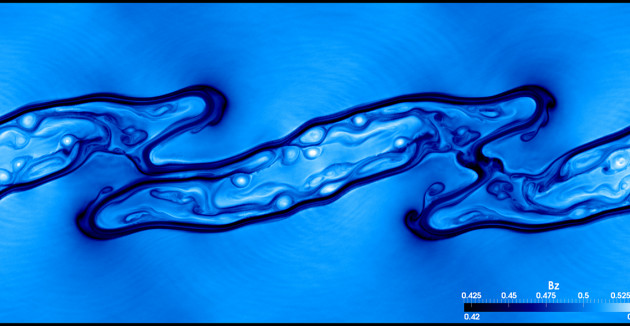
Catching Turbulence in the Solar Wind
Massive datasets plus modelling, visualization and analytics allow researchers to "see" the unseen: the turbulence in solar winds.
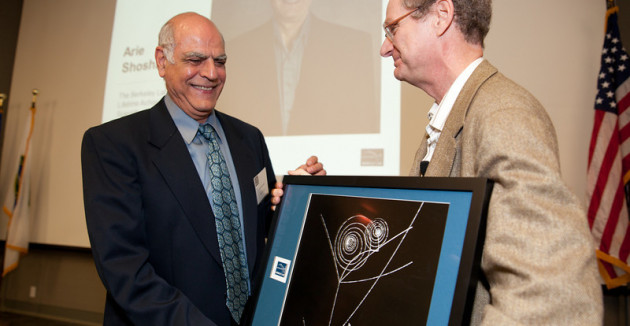
Arie Shoshani Earns Lifetime Achievement Award
More than 25 years ago, Arie Shoshani realized that researchers were facing significant challenges in organizing, managing and analyzing their scientific data. He set out to develop computer applicati…
The Scientific Data Management (SDM) group enables and accelerates scientific discoveries through effective data management and analysis tools and libraries. The SDM group’s research and development efforts focus on (1) scalable storage and I/O strategies, (2) autonomous data management infrastructure, (3) data life-cycle management, and (4) workflow optimization and automation. Our group actively works with data generation and analysis workflows to reduce the complexity of large scientific applications, including complex real-time workflows that could drive the next generation of scientific user facilities. Members of the SDM group work closely with application scientists throughout the DOE community, academic and industry researchers around the world.
Interim Group Leader: Alex Sim